Empirical Wavelet Transforms-based Feature Extraction of Vibroarthrographic Signal for Knee Osteoarthritis Detection and Stage Determination
Received Date: August 17, 2024 Accepted Date: September 17, 2024 Published Date: September 20, 2024
doi: 10.17303/jber.2024.8.201
Citation: Chaltu Dadi Tufa, Kinde Anlay Fante, Tewodros Belay Alemneh, Emnet Kebede Worassa (2024) Empirical Wavelet Transforms-based Feature Extraction of Vibroarthrographic Signal for Knee Osteoarthritis Detection and Stage Determination. J Biomed Eng Res 8: 1-18
Abstract
Background: Knee Osteoarthritis (KOA) is a degenerative joint disease that causes the knee joint cartilages to thin and the surfaces of the joint to become rougher, resulting in bone-on-bone contact, inflammation, and the formation of bone spurs, leading to pain, stiffness, so that the knee not able to move as the expected. The degradative progression eventually causes irreversible destruction of the articular cartilage and other tissues of the joints. Various imaging and signal-based techniques are used to diagnose KOA. However, the imaging modalities can capture only the gross cartilage defects but are not used to recognize subtle changes and for early detection of cartilage pathologies also due to their expensive, and time consuming, a simple and cost-effective method needs to be developed. The aim of this study is to develop a robust method for knee osteoarthritis detection using features extracted by empirical wavelet transform from vibroarthrographic signal and stage determination.
Methods: An acoustic signal was recorded be designing an electronic stethoscope using SG electret microphone and single-headed stethoscope to optimise joint sound detection from both healthy and knee osteoarthritic subjects with age limit above 45 years with symptoms of pain, stiffness, crepitus, and functional limitation. The recorded signals were pre-processed using a fourth-order low-- pass filter to remove noise and artifacts. Signal decomposition was performed using empirical wavelet transform (EWT) to extract audio features. An ensemble machine learning model was trained and tested using the extracted features.
Result: An ensemble machine learning model with an accuracy of 90.00% was obtained to detect four stages of KOA.
Conclusion: The results indicate that an ensemble machine learning model performed better for the detection of KOA at an early stage.
Keywords: Detection; Osteoarthritis; Vibroarthrographic Signal; Ensemble Machine Learning; Empirical Wavelet Transform
Introduction
The knee joint predominantly provides body support, and then enables bending motions during walking, due to these, it is prone to illness and wear and tear [1]. A healthy knee joint can bend and straighten because of a strong, flexible material and smooth slippery tissue called articular cartilage which protects, covers, and cushions bones against impacting each other during movement and it also allows the bones to glide over each other as the knee bends and straightens [2,3]. Knee joint disorder is a common musculoskeletal disease that arises as a result of ageing, injury, or repeated stress on the knee [4,5]. Osteoarthritis (OA) is the most widespread chronic rheumatic knee joint disorder which is characterized by the breakdown of articular cartilage [6]. According to the World Health Organization, about 595 million people worldwide were living with osteoarthritis in 2020, an increase of 132·2% since 1990 [7]. KOA is a degenerative joint disease that causes the knee joint cartilages to thin and the surfaces of the joint to become rougher, resulting in bone-on-bone contact, inflammation, and the formation of bone spurs, leading to pain, stiffness, so that the knee not able to move as the expected. The prevalence of KOA is rising because of the growing ageing of the population in developed and developing countries in addition to an increase in risk factors leading to KOA [8].
Different researchers developed many methods that are used in identifying the presence of knee joint disorder (abnormality of knee joint) using imaging technologies [9-11]. The study in [9], suggested a method that detects the early stage of articular cartilage degeneration using quantitative MRI and provides a direct measure of cartilage compression strain using a combination of MR imaging and site-specific 3D modelling of the subject's cartilage. Another researcher developed an automated decision support system for the detection and prediction of OA based on TB texture regions selected on knee and hand radiographs to identify pre-radiographic OA bone changes in addition to differentiating between OA and healthy joints [10]. Vibration signals obtained from the knee joint during flexion or extension movement can be used for detection of articular cartilage damage. As stated in [12], miniature accelerometers were attached to the patient’s skin using double-sided adhesive tape in the region of the knee corresponding to the lateral condyle of the tibia (LCT). The empirical mode decomposition (EMD) and Hilbert spectral analysis technique was used for feature extraction and an accuracy of 85% was obtained [12].
KOA is a musculoskeletal disease characterised by cartilage degeneration. Sometimes people do not aware that they are exposed to knee joint disorder because the disease could not indicate any known symptoms (like pain and bone spur) until it comes to the severity stage. Various imaging and signal-based techniques are used to diagnose KOA. However, the imaging modalities can capture only the gross cartilage defects but are not used to recognize subtle changes and for early detection of cartilage pathologies also due to their expensive, and time consuming, a simple and cost-effective method needs to be developed. Hence, this study is important in detecting the abnormality of the knee joint before it reaches its irreversible (severity) stage.
Method
An electronic stethoscope was constructed to collect data from both health subjects and patients with KOA of different stages. The second step was pre-processing of vibroarthrographic signals utilizing different filters to remove unwanted signals from the original signal. Then, feature extraction and feature selection were taking place using the selected method. Finally, the selected feature was fed to the classifier for training and classified into their stages using both deep learning and machine learning. Figure 1 shows the general block diagram of the method used in this study.
Designing of Electronic Stethoscope
Electronic stethoscope also called digital stethoscope takes the physical vibrations of sound, translates them into an electronic signal and converts the acoustic sound waves obtained through the chest piece into electrical signals which can then be amplified for optimal listening to improve diagnosis [13,14]. This device was constructed by using electret (condenser) microphone, single headed stethoscope, microphone cable and stereo plug which are locally available.
The first device used is the microphone. Microphone is an instrument which converts sound waves into electrical energy variations which may then be amplified, transmitted or recorded. An electret microphone, also called electret condenser microphone, is a type of electrostatic capacitor-based microphone with frequency of 1-20Khz. It eliminates the need for a polarizing power supply by using a permanently charged material [15]. This device is selected based on that electret microphone acquired higher quality knee joint signal than contact microphone [16]. Figure 2 shows the SG electret microphone used in this study.
The second component is a single head stethoscope. Stethoscope is a medical instrument used in listening to sounds produced within the body like heart, lung, and also sound produced in knee joint. Stethoscope chest piece (head) could be classified as dual head and single head. Single head stethoscope is preferable for designing electronic stethoscopes due to its wide frequency range and clear sound quality [13]. Figure 3 shows a full single head stethoscope (a) and the separated head of the stethoscope (b) used in this study.
In designing the electronic stethoscope, a connector (like a tube which connects the microphone sensor with the stethoscope), stereo plug and microphone cable is used to transmit sound signal from the sensor to the computer through software. The microphone welded with the microphone cable as shown in figure 4 (a). The other end of the cable was welded with a stereo plug, which will be connected to the laptop as shown in Figure 4 (b).
Finally, the sensor is connected with the head of the stethoscope using a connector, which is depicted in Figure. 5, resulting in the designed electronic stethoscope.
Data Collection
The study was conducted in Yordanos Hospital in Addis Ababa and Beza clinic in Adama. The data for this study was collected from 40 knee OA participants (16 males and 24 females, age between 45 and 71) and 25 healthy subjects (with age ranges 45 up 60) who have no history of any arthritis, knee injury and other knee related disease. MATLAB R2019b software was used in this study.
Inclusion Criteria
Knee osteoarthritis participants were subjects who had undergone knee x-ray or MRI and graded according to kellgren-Lawrence (KL) scores. KL scores is commonly used in clinical research, radiology reports, and medical practice to standardize the assessment and communication of knee osteoarthritis severity based on radiographic findings such as joint space narrowing, osteophyte formation (bone spurs), and other signs of degenerative changes in the joint. The KL grading system categorizes the progression of knee osteoarthritis into five stages. The grades range from 0 to 4, with each grade representing a different level of severity.
Grade 0: No detectable signs of osteoarthritis
Grade 1: Doubtful minimal osteophyte formation
Grade 2: Definite osteophyte formation and possible joint space narrowing
Grade 3: Moderate osteophyte formation, joint space narrowing, and some sclerosis (hardening) of the bone
Grade 4: Large osteophyte formation, severe joint space narrowing, and significant sclerosis
Experiment Setup
Subject Preparation
Subjects were informed about the experimental procedure and consented to confidentiality before the setup. Then skin preparation was performed by using ultrasound gel to decrease the friction between electronic stethoscope and knee joint skin. Adhesive tape was used to mount the sensor on skin. Participants were seated on a rigid chair, with both of his/her legs suspended. Then, an electronic stethoscope was attached to the subject’s skin at the mid-- patella position of the knee joint. The signal was recorded during movement of the leg from approximately 0 degree (full extension) to 90 degree of swinging leg with each flexion-extension cycle in 3-5 sec as shown in the Figure 6 (b) and (c) respectively.
Data Pre-processing
A total of 650 dataset of knee joint signals were obtained. The data contains five stages of knee joint signal such as Healthy (normal), Minor, Mild, Moderate and Severity. Figure 7 demonstrates knee joint signal obtained from all classes. The total number of dataset obtained for all classes is shown in Table 1 below
Then the signal filtered using low-pass butterworth IIR (Infinite Impulse Response) to remove high frequency noise above 1000 Hz as shown in figure 8. Additionally, notch filter was used to remove 50/60 Hz power line interference.
After filtering the data, the dataset was trimmed in to train, validate and test dataset, with a ratio of 60%, 20% and 20% respectively. Figure 9 (a) shows the obtained trained dataset before applying data augmentation technique. Finally, the training dataset was balanced and prepared for the next step of future extraction as shown in figure 9 (b).
Machine learning algorithms learn poorly when one class dominates another. Therefore, class balancing was performed in the training dataset during augmentation. Audio data augmenter (speed up, time shifting, volume gain, and add noise) has been used to increase the number of train dataset for the classification. Figure 10 shows the data augmentation performed in the training dataset.
Feature Extraction
Once pre-processing of the signal was done then, the next step was feature extraction. Vibroarthrographic signal is nonlinear and non-stationary. This makes it more difficult for the analysis.
Eventhough different studies have been done on vibroarthrographic signal decomposition and feature extraction for further classification, each of them has their own drawbacks. For instance, empirical mode decomposition and its variants have been used for VAG signal decomposition and feature extraction. The drawbacks of the EMD method include, mode mixing and lack of mathematical theory, permitting residual noise in the reconstructed signal is one of the limitations of ensemble empirical mode decomposition(EEMD). The other technique for breaking down and extracting features from vibroarthrographic signals is wavelet decomposition, but it cannot eliminate the noise in the different frequency bands of the signal [61]. In addition, wavelet decomposition has a fixed basis function, and this limits its application [53].
Empirical wavelet transform (EWT) is a flexible and adaptable signal process method that could solve this problem more than other signal decomposition technique like EMD and wavelet decomposition [70]. The EWT is a time-frequency method that is well suited to distinguish components containing varying frequency information in complex signals using adaptive wavelets as described below [70].
Steps for EWT
Step1.Compute the frequency spectrum of the signal by applying Fourier transforms.
Step 2. Divide the frequency spectrum into M consecutive segments.
- Modify the frequency range accordingly. The frequency spectrum range of the original signalft is converted to [0,π].
- Determine the local maxima. We assume that the number of local maxima is K.
- Find the frequency boundaries wM. M continuous segments include M+ 1 boundary, which include w0=0 and w0,π. That means we need to find M-1 extra boundaries. If K is greater than or equal to M, we arrange all local maxima in descending order and regard the first M-1 local maxima as boundaries (excluding 0 and π), otherwise we reset M according to the number of local maxima. Then we can obtain M continuous segments, each segment can be represented as
Step 3: Construct band-pass wavelet-based filters. To construct a band-pass filter in each segment there are two things that are needed. These are: empirical scaling function and wapvelet function which are designed below:

otherwise

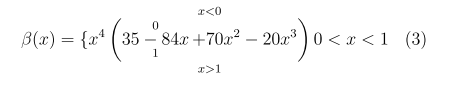
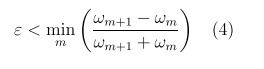
Step 4: calculate details and approximate coefficients The detail coefficients wf(f,t) can be obtained by inner product of the original signal and wavelet functions. And the approximate coefficient wf (0,t) can be obtained by inner product of the original signal and empirical scaling function φ1 (t).


Where φ1 (T-t) and ∀m (T-t) represent the conjugate complex numbers of φ1 (T-t) and ∀m (T-t) respectively.
Step 5: calculate the EWFs (empirical wavelet functions) (Determine empirical wavelet function (EWFs).
The approximation sub-signal f0 (t) and detail sub- -signal f1(t)


Classification Using Ensemble Machine Learning
Machine learning involves predicting and classifying data and to do so we employ various machine learning algorithms according to the dataset. Ensemble machine learning is among the machine learning models which give better performance for this study
Ensemble machine learning is a technique of combining multiple models to improve the overall performance of a machine learning task. The basic idea behind ensemble learning is that by combining the predictions of multiple models, we can often achieve better performance than any individual model.
The concept of ensemble learning dates back to the 1990s, when researchers began exploring the idea of combining multiple models to improve performance. Ensemble learning can be used for a variety of machine learning tasks, including classification, regression, and clustering. One of the earliest methods was bagging (bootstrap aggregating), which was introduced by Leo Breiman in 1996.
Bagging involves training multiple instances of the same model on different subsets of the data, and then combining their predictions [70].
Result and Discussion
Result
Feature Extraction
Signal decomposing using empirical wavelet transform
The initial step of feature extraction using Empirical Wavelet Transform (EWT) involved decomposing the vibroarthrographic signal to eliminate the highest frequency component present in the signal, which represents unwanted noise. Figure 13 illustrated signal decomposed utilizing EWT.
Signal Reconstruction
Once the signal was decomposed, the energy of each decomposed signal was computed. The empirical wavelet function (EWF) exhibiting the highest energy was then chosen for feature extraction. This selection was based on the understanding that the EWF with the highest energy has the same feature with the original signal and possesses similar features. It was seen from the energy calculation of both detail signal and approximate signal energy of approximate signal is higher than energy of detail signal. Additionally, it is understandable that an approximate signal contains high energy or important information of the signal, therefore feature extraction from the approximation signal may
Audio Feature Extraction
The vibroarthrographic signal was processed to obtain 29 audio features from both the time and frequency domains. These includes skewness, kurtosis, Peak2rms, variance, Shape Factor, Peak Value, Crest Factor, Clearance Factor, RMS, Mean, SNR, Standard deviation, Impulse Factor, peak frequency, Spectral Centroid, Spectral Decrease, Spectral Entropy, Spectral Flatness, Spectral Flux, Spectral Kurtosis, Spectral Roll off Point, Spectral Skewness, Spectral Slope, Spectral Spread, Energy, Entropy, Waveform length, Zero-crossing, and Auto-correlation.
Table 2, demonstrates values of extracted features using audio feature extractor. So, this study also selected this method to select the best features from 29 features extracted which include Peak frequency, Peak2rmsm, Peak value, kurtosis, Snr, spectralskewness, Mean, Entropy, Crest factor, Spectral Entropy.
Model selection
In the final phase of this study, various machine learning models were trained and assessed to identify the most suitable one for addressing the given problem. The evaluated models included support vector machine (SVM), Ensemble classifier, K-Nearest Neighbor (KNN), and long short-term memory (LSTM). The results of this analysis are presented in Table 3, which allows for a direct comparison of the performance of these different methods. The other task is finding the best hyperparameter values for a specific machine learning algorithm which is called hyperparameter tuning and it was done by applying bayesian optimization as a result of manual tuning is time consuming and grid search is computationally demanding and time-consuming.
In order to comprehensively evaluate the performance of the classification models, confusion matrix was employed. Confusion matrix is a valuable tool for evaluating the classification accuracy of a model. It provides a breakdown of the model's predictions, including true positives, true negatives, false positives, and false negatives. By examining the confusion matrix, we can gain insights into how well each model performs in terms of correctly classifying samples from different classes. In multiclass classification, a confusion matrix is a tabular representation that summarizes the performance of a model by displaying the counts of true positives (TP), true negatives (TN), false positives (FP), and false negatives (FN) for each class. The rows of the confusion matrix correspond to the actual labels of the examples, while the columns correspond to the predicted labels. By examining the confusion matrix, we can assess the model's strengths and weaknesses for different classes and better understand its overall classification performance.
Discussion
Knee osteoarthritis is a common degenerative joint disorder that impacts a large number of people globally, leading to considerable pain, functional limitations, and diminished quality of life. Precise identification and classification of knee osteoarthritis are vital for effective treatment strategies, disease control, and monitoring patient progress. While conventional diagnostic techniques like X-ray and Ct-scan provide valuable insights into the structural aspects, they often overlook the dynamic characteristics of the condition but often fail to capture the dynamic nature of the disease. Consequently, there is a demand for alternative methods that can provide a more comprehensive and functional evaluation of knee osteoarthritis.
One of the key focuses of this study was the method of feature extraction, which plays a crucial role in signal processing and forms the foundation for machine learning. In the literature review (Section 2.4), several signal decomposition methods were explored, including continuous wavelet transforms, empirical mode decomposition (EMD), ensemble empirical mode decomposition (EEMD), and empirical wavelet decomposition. Each of these methods has its own advantages and drawbacks.
For instance, empirical mode decomposition decomposes signals into their intrinsic mode functions (IMFs), and the reconstruction of these decomposed signals provides the original signal. However, this method lacks a solid mathematical theory, and it tends to decompose signals into numerous intrinsic mode functions, resulting in frequency overlap. Consequently, it was not preferable for this study. Instead, this study employed the empirical wavelet transform (EWT), which is derived from the combination of empirical mode decomposition (EMD) and wavelet transform. The empirical wavelet transform adaptively decomposes non-stationary signals into empirical wavelet functions (EWFs) by segmenting the Fourier spectrum of the signal. This approach offers an advantageous alternative for feature extraction in this study, as it addresses the limitations of EMD and provides a more effective decomposition of signals.
The feature extraction process involved extracting a wide range of signal characteristics including skewness, kurtosis, Peak2rms, variance, Shape Factor, Peak Value, Crest Factor, Clearance Factor, RMS, Mean, SNR, Standard deviation, Impulse Factor, peak frequency, Spectral Centroid, Spectral Decrease, Spectral Entropy, Spectral Flatness, Spectral Flux, Spectral Kurtosis, Spectral Roll off Point, Spectral Skewness, Spectral Slope, Spectral Spread, Energy, Entropy, Waveform length, Zero-crossing, Covariance, and Auto-correlation.
However, to reduce the dimensionality of the feature set and improve the efficiency of the classification model, the FSC-MRMR method was employed. This method utilizes a Minimum Redundancy Maximum Relevance (MRMR), to identify the most informative and discriminative features.
After applying the FSC-MRMR method, only 10 features were selected for further analysis. These features were peak-frequency, peak2rms, peak value, kurtosis, SNR, spectral skewness, mean, entropy, crest-factor, and spectral entropy. These 10 features were deemed to have the highest relevance and discrimination power in characterizing the signals under investigation.
By employing the FSC-MRMR method, we were able to effectively reduce the feature space while retaining the most informative features. This not only improved the efficiency of our classification model but also ensured that the selected features were highly relevant in capturing the essential characteristics of the signals.
This study employed a combination of deep learning (Long Short-Term Memory) and machine learning techniques like, Multi-class SVM, k-nearest neighbour (KNN), and an ensemble classifier. After comparing their performance, it was found that the ensemble classifier achieved the highest accuracy among the two models. This indicates that the ensemble classifier was able to effectively classify the data and make accurate predictions for the given problem and we successfully classified knee osteoarthritis into five distinct classes, including a healthy/control group.
Conclusion
Vibroarthrographic signals (Knee joint sound) were recorded using self-made, portable, and easily accessible electronic stethoscopes during the flexion and extension of participant leg in the sitting position at 90 degrees. After preprocessing, the signal was decomposed into its different frequency component (filter banks) based on the boundary obtained from Fourier segment using Empirical wavelet transform (EWT).
As seen on the result part from the ROC curve, AUC values of each class are above 0.9 and this implies that the classifier is able to distinguish between positive and negative examples with a high degree of accuracy. Additionally, this thesis showed that the ensemble machine learning model proved to be highly effective in accurately classifying samples, as revealed by the precision score of 96.34%, recall score of 97.53%, and f1_score of 96.93%. Furthermore, the sensitivity and specificity metrics of 97.53% and 92.86%, respectively, demonstrate the method's ability to correctly identify positive and negative instances.
Finally, it is commonly accepted fact that loading like walking downstairs and upstairs has different effect on knee joint signal because it causes an increase in the friction between the femoral and tibia bones at the end of the joint, resulting in a change in the signal's characteristics. Therefore, for the future data collection, it is recommended to use wearable devices for continuous monitoring or wireless sensors to collect signals in different position and situation. It is also advisable to integrate the method into clinical practice because vibroarthrographic signal is the promising method for early detection of knee osteoarthritis.
Declarations
Ethics Approval and Consent to Participate
An ethical approval was obtained from the Institutional Review Board of the Jimma University Institute of Health Science. All methods were carried out in accordance with the ethical standards established in the 1964 Declaration of Helsinki and its later amendments or comparable ethical standards.
Consent for Publication
Informed consent was not obtained; thus, the Jimma University Institute of Health Science ethical review committee waived this requirement. Participants' anonymity and privacy were upheld, and the data were sufficiently anonymized.
Availability of Data and Materials
The datasets used during the current study are available from the corresponding author upon reasonable request.
Competing Interests
The authors declare no competing interests.
Funding
The author received no financial support for the research, authorship, and/or publication of this article.
Authors' Contributions
All the authors made significant contributions to the work reported, whether by conception, study design, execution, acquisition of data, analysis and interpretation, or in all these areas; by drafting, revising or critically reviewing the article; by giving final approval of the version to be published; by agreeing on the journal to which the article has been submitted; and by agreeing to be accountable for all aspects of the work.
Acknowledgements
Yordanos Hospital in Addis Ababa and Beza clinic provided material needed for this study
- M Kloppenburg, F Berenbaum, (2020) “Osteoarthritis year in review 2019: epidemiology and therapy,” Osteoarthr. Cartil, 28: 242-8.
- R Gong, K Hase, H Goto, K Yoshioka, S Ota, (2020) “Knee osteoarthritis detection based on the combination of empirical mode decomposition and wavelet analysis,” J. Biomech. Sci. Eng., 15: 3.
- F Flandry, G Hommel, (2011) “Normal anatomy and biomechanics of the knee,” Sports Med. Arthrosc., 19: 82-92.
- SL Kolasinski et al. (2019) American College of Rheumatology/Arthritis Foundation Guideline for the Management of Osteoarthritis of the Hand, Hip, and Knee,” Arthritis Care Res., 72: 149-62.
- D Huang et al. (2018) “The Diagnosis and Therapy of Degenerative Knee Joint Disease: Expert Consensus from the Chinese Pain Medicine Panel,” Pain Res. Manag., 2018.
- B Snoeker et al. (2020) “Risk of knee osteoarthritis after different types of knee injuries in young adults: A population-based cohort study,” Br. J. Sports Med., 54: 725-30.
- O Collaborators (2023) “Articles Global, regional, and national burden of osteoarthritis, 1990 – 2020 and projections to 2050 : a systematic analysis for the Global Burden of Disease Study 2021,” 5: 3.
- C Palazzo, C Nguyen, MM Lefevre-Colau, F Rannou, S Poiraudeau (2016) “Risk factors and burden of osteoarthritis,” Ann. Phys. Rehabil. Med., 59: 134-8.
- S Nebelung et al. (2019) “Detection of Early-Stage Degeneration in Human Articular Cartilage by Multiparametric MR Imaging Mapping of Tissue Functionality,” Sci. Rep., 9:1-11
- GW Stachowiak, M Wolski, T Woloszynski, P Podsiadlo, (2016) “Detection and prediction of osteoarthritis in knee and hand joints based on the X-ray image analysis,” Biosurface and Biotribology, 2: 162-72.
- Antony K. McGuinness K Moran, a NEO’Connor (2017) “Automatic detection of knee joints and quantification of knee osteoarthritis severity using convolutional neural networks,” arXiv.
- JC Chen, PC Tung, SF Huang, SW Wu, SL Lin, KL Tu (2013) “Extraction and screening of knee joint vibroarthrographic signals using the empirical mode decomposition method,” Int. J. Innov. Comput. Inf. Control, vol. 9, no. 6, pp. 2689–2700, 2013.
- YT Cheng, CC Tai, W Chou, ST Tang, JH Lin (2018) “Analyzing the audio signals of degenerative arthritis with an electronic stethoscope,” Rev. Sci. Instrum., 89: 8.
- KS Kim, JH Seo, CG Song (2012) “An acoustical evaluation of knee sound for non-invasive screening and early detection of articular pathology,” J. Med. Syst., 36: 715-22.
- C Teague (2016) “A Robust System for Sensing Acoustic Emissions for Wearable Knee Health Assessement by Copyright © 2016 by Caitlin Teague. A Robust System for Sensing Acoustic Emissions for.
- A Łysiak, A Froń, D Baoczkowicz, M Szmajda (2019) “The new descriptor in processing of vibroacoustic signal of knee joint,” IFAC-PapersOnLine, 52: 335-40.
- LG Kabari, UC Onwuka (2019) “Comparison of Bagging and Voting Ensemble Machine Learning Algorithm as a Classifier,” Int. Journals Adv. Res. Comput. Sci. Softw. Eng., 9: 19-23.
Tables at a glance
Figures at a glance