Oil Extraction from Allanblackia Floribunda Seeds and its Optimization via Response Surface Methodology
Received Date: August 24, 2024 Accepted Date: September 24, 2024 Published Date: September 27, 2024
doi: 10.17303/jmsa.2024.8.201
Citation: Balogun TA, Woyengibunugha E, Adepoju TF (2024) Oil Extraction from Allanblackia Floribunda Seeds and its Optimization via Response Surface Methodology. J Mater sci Appl 8: 1-10
Abstract
In this study, the seed oil was extracted from Allanblackia floribunda seed and to model and optimize the process condition of oil extraction, response surface methodology was used. Physicochemical properties of the oil were carried in order to determine the suitability of the oil for industrial applications. the seeds were collected from Oyigbo in Etche Local Government Area of Rivers State, Nigeria. N-hexane was used as solvent for the extraction; the experiment was designed using Box-Behnken Design approach on three levels, three factors which generated 17 experimental runs. Independent factors considered were time of extraction (X1), volume of solvent (X2), and weight of sample (X3). The accuracy of the regression model obtained from the optimization software was determined using the regression coefficient.
Results showed the Allanblackia floribunda seed was rich in oil with the predicted optimum seed oil yield of 49.04% (wt./wt.) was established at the optimal condition of extraction time 50.74 min, sample weight 50.00 g and solvent volume 299.99 mL. The optimized condition was validated with the actual seed oil yield of 48.90% (et./wt.). The predicted values were well within the range. The coefficient of determination (R2), which determines the model accuracy was formed to be 99.97% while the R2 (adj) was 99.93%, respectively. These values revealed that this regression was statistically significant and therefore, it could be used in theoretical predication of the oil extraction, the study concluded that Allanblankia floribunda seed are found to be rich in oil, with high saponification value and low iodine value and can be useful in many industries as raw material for products formation.
Keywords: Allanblackia Floribunda Seeds; Response Surface Methodology; Process Optimization; Oil Extraction; Physicochemical Properties; Regression Parameters
Introduction
On a worldwide scale, the supplies and consumption of oils and fats have generally been described in terms of 17 commodity oils, four that originate from animals and thirteen that are derived from plants, namely soybean, palm, rape/canola, sunflower, coconut, palm kernel, cottonseed, groundnut (peanut), olive, corn, sesame, rice bran, and flaxseed oil.
The use of oil in industries for production of cosmetic, biofuel, bio lubricant and other products has become greatly increase, which has necessitated the need to search for alternative source through biomass (Agricultural on seeds) to meet up with the needs of the incessant increase in the demand for oil by both domestic and industries have resorted in search for using underutilized seeds as sources of oil supplement the already current traditional source of oil (Ugbogu et al., 2014).
Allanblackia fioribunda seed is one of the underutilized seed oil with bioactive component.additionally, few studies have specifically focused on the roles of these phytochemical in preventive strategies or as pharmacological agents used in the treatment of chronic diseases.
Generally, the extraction of oil from the seeds can be achieved via various methods such as mechanical pressing, (Joglekaw and May, 1987; Haidur and Pakshirahaj, 2007; Schinas et al., 2009) pressurized solvent extraction (Megiuely, 1991), soxhlet extraction (Meher, et al., 2006), Ultrasonic extraction (Ramos et al., 2009; Rodriguez et al, 2012), Agueous Enzymatic oil extraction (AEOE) to mention but few (Perez-Serradilla et al., 2002).
Mechanical pressing is the oldest and easiest method used widely but the oil produced always with low value meanwhile (Betiku and Adepoju, 2013) reported the use of supercritical CO2 extraction. The oil yield obtained was higher than what was obtained from using solvent extraction and of high purity but the high operating and investment cost make this method not suitable and investment cost make this method not suitable to be used. solvent extraction has various advantage including high yield use turbidity, environmentally friendly and cost effective [1] which was why solvent extraction of oil from Jatropha cursus was carried out by Kian et al. (2012) while Umer et al. (2011) studied the solvent extraction of oil from Moringa Olifera. Betiku et al. (2012) extracted oil from sorrel seed using solvent extraction while solvent extraction of oil from chrysophyllumn albinism oil seeds and its quality characterization was carried out by [1]. In other study, Betiku et al. (2012) worked on solvent extraction of oil from Beniseed (Sesamum indicum) oil seed.
This work therefore focused on the possibility of using Allanblackia floribunda seed for oil production. for modeling and process optimization, response surface methodology (RSM)were employed so as to generate the number of experimental runs, determine the predicted yields, compare the error values and analysis the various variable factors responsible for optimum production of oil so as to increase the process effciency. Lastly the properties of allanblackia seed oil were evaluated with a view to determining its suitability as non-drying and edible oil.
Materials and Methods
Materials
The allanblackia floribunda seeds used in this work was collected from Oyigbo in Etche Local Government Area of Rivers State Sustainable Development Agency (RSSDA). The seeds used for the study were removed from ripe mature fruits manually through maceration. The extracted seeds were dried in the sun for three days until a constant weight was obtained. Finally, the cleaned seed were grinded into powder and all the chemical used in this study are of analytical grades.
Methods
Experimental Design
To optimize the oil extraction from Alanblackia floribunda seeds powder, three level – three factors were considered Box-Behnken Design (BBDRSM) was employed which generated 17experimented runs used to study the effects of selected factors on oil yield, Table l showed the selected factors which are extraction time (X1), solvent volume (X2) and the sample weight (g) (X3) and their levels. Th ese factors were also used for RSM modeling and optimiza tion. For the coeffcient of the quadratic model of the response settings, multiple regression models was adopted using design expert software 6.0.3.1 to evaluate and determine the coeffcient of the full regression model equation and their statistical significance.
Descriptions of oil extraction procedure
Based on the experimental design by Box Behnken design, seventeen experimental runs were generated and were carried out, soxhlet extractor was used for the extraction process; the powdered sample was weighed and place in the extractor through the muslin cloth placed in a thimble of the apparatus, n-hexane was used as organic solvent for extraction. their level three factors were considered, the excess n-hexane was recovered using rotary evaporator and the yield of the oil was obtained using e.g. (1)

Physicochemical analysis of the extraction oil
The properties of the oil were evaluated using standard of AOCS (2016) and offcial analytic method of analysis 16th edition, association of offcial analytical chemist Washington D. C. AOAC, (2016).
Variable interactive effects
To study the effects of independent variable on oil yield, three factors were taking into consideration (Table 1). The interactive effects of the variable on the oil yield were considered by allowing for the linear (X1, X2, X3), the interaction (X1X2 , X1X3 , X2X3 ), and the quadrati c (x12 x22 x32)on the response. The variable inter active effect lead to the formation of regression equation and explained vividly how the contour and three-dimensional plots are obtained.
Optimization of oil extraction
Important aspect of any regression analysis is to confirm the simple linear regression model is satisfied. The relationship between the response variable (oil yield) and selected variables (X1, X2, X3), are assumed to be in form of linear, interaction and quadratic.
To check the model accuracy and the model estimation capabilities, the coefficient of determination (R2 ) was determined using e.g. (2). Their values were used together by comparing the evaluated values for the models.
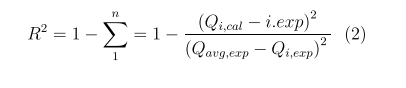
Where Øi,exp is the experimental value, Øi,cal is the calculated value and Øavg.exp is the average experimental value.
Results and Discussion
Optimization Study: Experimental oil yield and predicted data
Table 2a depicts the actual factors considered in
this study with experimental results, and the predicted values obtained. Design Expert 6.0.3.l software was employed
to evaluate and determine the coefficients of the full regression model equation and their statistical significance. Table
2b showed the results of significance for every regression coefficient. The results showed that the p-values of the model
terms were significant, i.e. p< 0.05. In this case, the linear
terms, the two cross products (X1X3 and X2X3), and the three quadratic terms were all remarkably
significant model terms at 95% confidence level except X1X2
with f-value = 2.50; p-value >0.05. In order to minimize error, all the coefficients were considered in the design. Table
2c shows the analysis of variance of regression. The data obtained fitted best to a quadratic model and the linear terms
were well interactive to exhibit low standard error. Furthermore, the coefficient of determination (R) was found to be
99.97% while the R2
(adj) was 99.93% with low standard deviation of 0.0633 and mean average of 42.22, respectively.
Meanwhile, all p-value coefficients were less than 0.1, which
implied that the model proved suitable for the adequate representation of the actual relationship among the selected factors. These values revealed that this regression was statistically significant; only 0.045% of total variations were not explained by this regression model. Therefore, it could be
used in theoretical prediction of the oil extraction.
Modeling equation
The final equation in terms of actual factors in quadratic term is expressed in Eq. (2).

A graph can provide a kind of visual method to observe responsive value and to test parameter level relation. Figure 1 shows the relationship between the predicted and actual experimental results obtained. Graphical observation indicated that the predicted values were in line with the experimental results and the prediction values were so good.
Figure 2a shows the contour and the response surface plots representing the effect of extraction time, solvent volume and their reciprocal interaction on oil yield while keeping sample weight constant at zero level. The results showed that mid extraction time and high solvent volume favoured oil yield while viz-versa led to low oil yield (Figure 2a). The contour and response surface plots representing the effect of extraction time, sample weight and their reciprocal interaction on oil yield while keeping solvent volume constant is depicted in Figure 2b. The high sample weight and mid extraction time produced high oil yield whereas the reverse yielded only low amount of oil. The combination of high sample weight and low extraction time did not significantly increase the oil yield. However, the highest oil yield was observed at low sample weight and high extraction time. Figure 2c shows the contour and response surface plots representing the effect of solvent volume, sample weight and their reciprocal interaction on oil yield while extraction time constant at zero level. Highest oil yield was recorded at the highest sample weight and highest solvent volume. A decreased in level of oil yield was observed at low sample weight and high volume of solvent which give rise to the low volume of oil produced.
However, based on statistical solutions, a total of five (5) solutions were randomly selected by the RSM model, the solutions predicted that the highest conversion yield of Allanblackia oil was 54.2689% (v/v) at the following optimized conditions: sample weight of 50 g, solvent volume of 200 ml and extraction time of 40 min (Fig. 3c). Using these optimal factor values under experimental conditions in three independent replicates, an average content of 54.27% (v/v) was achieved, and this value is well within the range predicted by the model. This showed RSM is a good optimization tool in modelling and optimization of Allanblackia seed oil extraction when n-hexane was employed as solvent.
Physicochemical properties of Allanblackia floribunda oil
Table 3 shows the results of the physicochemical properties of the extracted (ASO) oilobtained using AOCS and AOAC (2016) standard methods. The oil obtained was darkyellowish in color with the moisture content of 0.16% and specific gravity of 0.89 showing thatthe oil is higher than water and will form an upper layer in a water-oil mixture.
The low acid value is usually twice as large as the FFA was obtained in this study and itindicated the good resistance of the oil to hydrolysis.
The peroxide value measures the content of hydroperoxides in the oil and it's how value 8.6 meg/kg indicates resistance to oxidation. High saponification value of 185.7 mg KOH/g with a low iodine value of 39 g/100 g indicated a low concentration of triglycerides and the oilcontained a substantial level of unsaturation. The iodine value determines the level of unsaturation, and the Allanblackia floribunda seed oil is classified as non-drying because the iodine value is less than 100 g/100g.
The low acid value 0.6 mg KOH/g oil ofthe seed oil showed that it is not only edible but could also have a long shelf life. A high saponification value of (185.7 mgKOH/g oil) suggesting high concentration of the triglycerides.
Conclusions
the study showed that Allanblackia floribunda seed is rich in oil. the work demonstratedresponse surface methodology is a good tool for determining the optimal condition for thesolvent extraction of oil from Allanblackia floribunda seed. From the design expert employed in this work, the predicted optimum seed oil yield of 49.04% (wt./wt.) was established at the optimal condition of extraction time 50.74 min, sample weight 50.00 g and solvent volume 299.99 mL. The optimized condition was validated with the actual seed oil yield of 48.90% (v/v). Physiochemical analysis of the allanblackia seed oil advocates it could serve as useful oil forfood industries and feed stock in many chemical industries.
Acknowledgements
Authors wish to thank the Laboratory staff of Food Science Technology and ChemicalEngineering Department of Rivers State University, Port Harcourt, Rivers State.
- Adepoju TF, A Abiodun (2013) Statistical approach to oils extraction from sorrelseed (Hibiscus Sabdariffa L.) and its quality characterization. International Journal ofScience and Engineering Research, 9.
- Adeniyi TS, Densley, HR Lemu (2014). Deforestation: FCT sits on atighttrope. Daily Trust, City news.
- AOAC (1990) Official methods of analyses of the association of official analyticalchemists. 15th Edn., Washington DC, USA: Association of Official AnalyticalChemists.
- Australian Academy of Science AAS, (2018). The science of climate change. Availableonline at: https://www.sci ence.org.auw/learning/generalaudience/science-booklets-O/science-climate-change/2-howhas-climate-changed.
- Balogun AT (2022) Extraction and Characterization of Nigerian Allanblackia Floribunda Oil Seed using Different Solvents for Biodiesel Production: PhD Thesis, RSU.
- Cyblug (2016) Climateand Weather. Abuja City, Nigeria. Available online at:www.abujacity.com/abuja and beyond/climate-and-weather.html.
- Decision Lens (2015) Decision Lens. Retrieved from http://www.decisionlens.com
- Dongjie G, Hidetoshi F, Weijun G, Kazuyuki W (2008) Land use change ofKitakyushu based on landscape ecology and Markov model. J Geogr Sci. 18: 455-68.
- Eletta OAA, SI Mustapha, OA Ajayi, AT Ahmed(2018) Optimizationof Dye Removal from Textile Wastewater using Activated Carbon from Sawdust.Nigerian Journal of Technological Development. 15: 26-32.
- Food and Agriculture Organisation (FAO): Water Reports (2010). The wealth of waste:The economics of wasteater use in agriculture.
- Intergovernmental Panel on Climate Change (IPCC). (2007). Assessment report; Climate Change 2007 Summary for policymakers. Valencia, Spain.
- Jonathan RBF, AA Eileen, PJ Dennedy- Frank, K Timm, MB Timothy (2017) Impact of satellite imagery spatial resolution on land use classification accuracy and modeled water quality
- Kahinda JMESB Lillie, AE Taigbenu, M Taute, RJ Boroto (2008) Developing suitability maps for rainwater harvesting in South Africa. Physics andChemistry of the Earth, 33: 788-99.
- Mahmoud SH, Alazba AA (2014) The potential of in- -situ rainwater harvestingin arid regions: developing a methodology to identify suitable areas using GIS-baseddecision support system Saudi Society for Geosciences, 1-13.
- Maimbo M, K Elizabeth, M Bancy, Alex, DB Tanguy, et al. (2005) Mapping the potentials of rainwater harvesting in Africa. GWP-APSeries ofICRAF publications, Nairobi.
- Mbilinyí BP, SD Tumbo, HF Mahoo, EM Senkondo, N Hatibu (2005) Indigenous Knowledge as Decision Support Tool in Rainwater Harvesting. Physics andChemistry of the Earth. 30: 792-8.
Tables at a glance
Figures at a glance